Insurance execs aren’t short on tools. They’re drowning in them. Claims automation dashboards, underwriting engines, chatbots, fraud detection layers, every vendor shows up with a polished demo and a slide deck full of ROI metrics. What they don’t show is the operations mess they leave behind.
Most AI implementation plans in insurance start in the wrong place. Someone in the boardroom greenlights a pilot. IT spins up a sandbox. A vendor integration begins. Internal teams get a new platform dropped into their lap with zero workflow alignment. Six months later, nobody uses it. Or worse, claims are stuck in triage purgatory, underwriting decisions get overridden manually, and the fraud triggers fire off false positives every other hour. Executives call it a failed experiment. The real failure was thinking this was a tech initiative.
AI doesn’t fix broken workflows. It exposes them. Every inefficiency you’ve managed to patch with headcount or spreadsheet gymnastics gets magnified under automation. That’s why ops-first strategy matters. Not tool selection. Not vendor speed. Not cost per seat. What matters is where friction lives inside the business, how decisions actually get made, and what blockers stand between your teams and better outcomes.
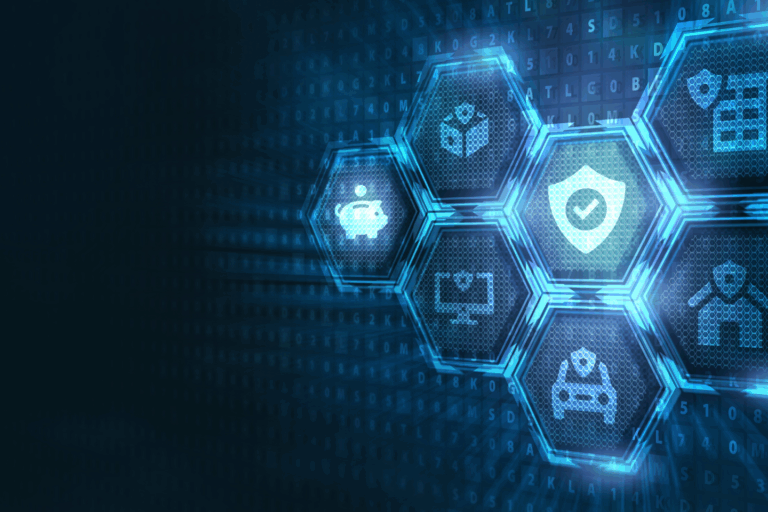
The Reality of AI Implementation
Claims Automation Challenges
Take claims automation. It’s the poster child of AI potential. Image recognition, document parsing, real-time video assessments, all technically impressive. But implementation falls apart when adjusters aren’t brought in early. When legacy policy systems don’t sync. When managers still escalate every non-standard case because they don’t trust the algorithm. You don’t need more AI. You need clarity on the claim lifecycle, process dependencies, and exception workflows. Automate that first.
Underwriting Obstacles
Underwriting’s no different. Everyone wants faster bind times and dynamic pricing models. But underwriting still lives inside Excel exports and tribal logic passed down from the senior analyst who retired two years ago. Machine learning can build risk models that outperform traditional scoring—but that’s not what stalls adoption. It’s ops friction. Data gaps. Regulatory complexity. Internal resistance to algorithmic overrides. AI isn’t failing. The organization just hasn’t mapped how underwriting decisions are made in the real world.
Fraud Detection Realities
Fraud detection sounds like a quick win. It’s not. You’ll catch more anomalies, sure. But unless your investigators can act on those signals, without jumping through three systems and calling IT for log access, fraud prevention stays stuck at theory level. AI can scan for doctored images and suspicious patterns in claims behavior. But if that output doesn’t translate into faster flagging, simpler escalation paths, and better loss ratio control, it’s just noise. Again, workflow first.
Where Implementation Breaks Down
Customer Service Automation
Customer service automations face the same wall. Chatbots, onboarding flows, sentiment analysis—they look clean in a vendor demo. But real call center operations aren’t structured for smooth handoffs. Teams still get dropped sessions. CRMs aren’t synced. Reps redo intake forms because the bot missed half the details. Customers notice. Satisfaction drops. You didn’t automate experience. You just automated friction.
Compliance Challenges
Compliance gets its own flavor of pain. AI can monitor transactions, validate customer data, even generate reports automatically. But most insurance firms still operate in a hybrid swamp of legacy mainframes, manual audits, and patchwork tech layers. You don’t get clean compliance unless your operational systems are clean. Otherwise, you’re just using AI to document the chaos.
The Core Problem
Here’s the deeper problem—most insurance firms still think of AI as an overlay. Something you bolt onto existing processes to get incremental lift. That model never works. You can’t bolt intelligence onto a process that wasn’t designed to be intelligent. You have to rework from the inside out. That’s where most implementation plans die—inside the gap between operational design and technical deployment.
The issue isn’t AI. It’s how AI gets framed. Every vendor sells the features. Few talk about the friction. Nobody explains what internal shift has to happen before any of this delivers value. The execs who get it right don’t start with tech. They start with workflows. With operational edge cases. With where claims stall, where fraud hides, where underwriting gets overruled, where service breaks down. They ask the only question that matters: where’s the real friction, and what’s the real cost of not fixing it?
This is not a tools problem. It’s a decision architecture problem. Insurance firms already have AI. What they don’t have is operational clarity. They’ve got dashboards with no adoption. They’ve got models with no integration path. They’ve got automation projects sitting half-finished because someone realized two months in that the core system can’t talk to the claims engine without a custom connector. That’s not a tech issue. That’s a planning failure.
The Way Forward
If you’re buying tools before you’ve mapped your decision chains, you’re setting yourself up to fail. Most automation roadmaps are just glorified software wishlists. There’s no process accountability, no behavioral alignment, no ops strategy. Just another shiny layer on a stack that’s already unstable. That’s how half-baked automation projects get sold in—and buried a year later when the outcomes stall.
The way forward isn’t more tools. It’s operational clarity. AI implementation in insurance only works when it’s built around how your teams actually work—not how your vendors wish they worked. Every successful project I’ve seen starts with brutal process analysis. What gets done, who touches it, where it breaks, and what decisions are being made under pressure. Then—and only then—do you decide what gets automated. And how.
If this sounds like your firm, you’re not alone. Most execs I speak to are in the same spot. They’ve got tech budgets, project plans, executive support. But they don’t have a roadmap that connects automation to real ops outcomes. They’ve got AI initiatives that sound great on paper and stall out on day 30.
You don’t need another tool demo. You need a strategy. A practical one. Built around your actual workflows, not a vendor’s feature set. That’s what we do.
Book a strategy call. Let’s fix the real problem.